
One production step follows the other, the different manufacturing processes interlock smoothly: What looks so simple in industrial production today takes enormous effort and a lot of experience. This is the only reason why mass-produced products can be manufactured economically today with zero or almost no defects. Developers and operators have an important role to play in this context. The University of Stuttgart’s Institute for Machine Tools (IFW) is looking how machining manufacturing systems could be made to optimize themselves.
Yes, a drilled hole is a drilled hole, but that is only superficially true. In fact, they can be quite different even if they were made by the same drill on the same machine. The drillings could be slightly elliptical or the roughness of their inner surfaces might differ. Even if the deviations are only in the range of a few thousandths of a millimeter, it could mean the difference between quality products and rejects in everyday industrial life. “The causes for these fluctuations can be manifold,” says Rocco Eisseler, Group Leader for Machining Technology at the University of Stuttgart’s IfW. “The speed or feed rate of the drill bit plays a role, as does the type of material the workpiece and drill bit are made of, but also factors such as cooling during drilling.” However you twist it, no two drilled holes are ever the same. And this also applies to any other machining technology, such as turning or milling.
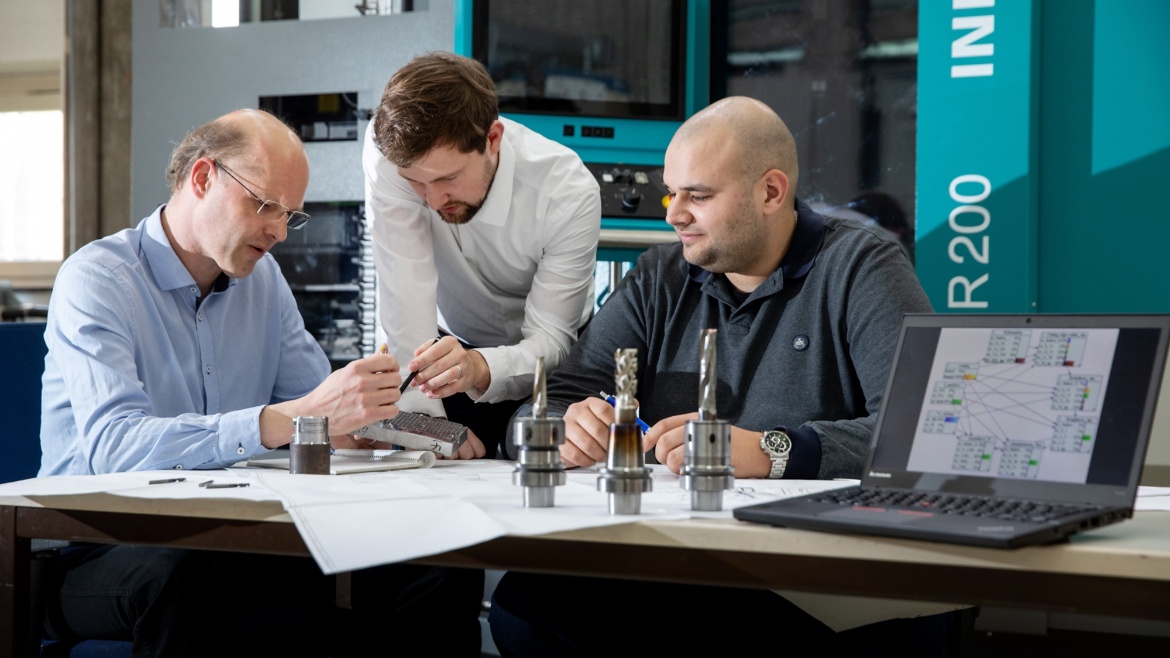
Working at the limits
Of course, the people who operate such production machines have the requisite knowledge and experience to produce a workpiece of the required quality within the specified tolerances,” says Eisseler. “However, based on the specifications of the tool manufacturers, it is usually necessary to adapt the machining parameters specifically to the respective machining processes, i.e. to always find the optimum.” For example, when it comes to very complex workpiece requirements or making manufacturing processes as economical as possible. “And there is always the issue that all these influencing variables also influence each other,” says the group leader. The hole may meet the geometric quality specifications at a certain drill speed and feed rate, but the drill bit may wear out relatively quickly. Not only would that necessitate an earlier replacement, but the wear would also lead to a lower quality result in terms of roundness and surface roughness.
So everything is connected to everything else."That’s why we chose drilling as an example for our research into how an expert system could be used to optimize the various demands of the manufacturing process,” says the scientist. This required a lot of drilling. However, the diameter of the drill and the material of the workpiece were always the same. “What varied were quantifiable parameters, such as speed and feed rate,” says Eisseler. It was then necessary to relate these input variables to the output variables of roundness and surface roughness, to which end, the team used a process called a Bayesian network. “Bayesian networks make it possible to establish a connection between cause and effect, even if there are no simple causal relationships,” explains Eisseler. “With them it is also possible to take account of the fact that wear on the drill bit affects both the input and output variables - Bayesian networks function in both directions, so to speak.” This is decisive in so far as, in addition to the correlations, causalities can also be discerned.
Bayesian networks make it possible to establish a connection between cause and effect, even if there are no simple causal relationships.
Rocco Eisseler
Interdisciplinary collaboration
The greater vision behind such research is that of a self-optimizing manufacturing system. IfW Director Prof. Hans-Christian Möhring explains: “Such a system would independently identify the optimization potential of a manufacturing process and initiate the necessary measures to realize this potential.” This could either be done automatically - an area of specialization in the IfW's research - or in interaction with the operator. “Currently, such a system remains aspirational,” says Möhring, “because the trained human operator can permanently record a wide range of information, intuitively anticipate the incipient states and virtually take them into account in real time during process control.” But with the help of machine learning, this will change in the future. “This could involve Bayesian networks, as in the case of the drilling process, but completely different methods, such as deep neural networks, are also conceivable,” says the engineer. The ultimate choice would depend on the specific task. “We work in interdisciplinary collaboration with other faculties within and from outside the university.”
Möhring's team takes a holistic approach to the self-optimizing manufacturing system, starting with the definition of the relevant machining process in the CAD/CAM programs and ending with the finished result, the quality of the workpiece and the wear and tear on the tool. “It is always important to trace the knowledge gained back to the process parameters,” emphasizes Möhring. “And that’s not easy, given the multiple, sometimes opposing, interdependencies.” The whole concept is still in its infancy in industry. “However, the manufacturers and users of the manufacturing systems are very open to the idea,” says Möhring. “Perceptions are changing, not least due to the buzzword ‘industry 4.0’.” Even if the vision of the self-optimizing manufacturing system is much older than the digitization of industrial production processes, he adds. “There are companies that have been collecting manufacturing data for many years. But for many, the question of how they might be able to use this data remains unanswered.” From his point of view, however, there is no single solution here, but only answers to each individual case.
Möhring also believes that there is no simple yes or no when it comes to the question of whether self-optimizing production systems should function automatically in the future or only in interaction with the operator. He illustrates this point with an analogy to autonomous driving: “Would you entrust yourself blindly to an autonomous vehicle at 160 kilometers per hour or would the car at least have to signal to you regularly and comprehensibly that it has everything under control,” he asks - and it sounds rhetorical.
Michael Vogel
Dipl.-Ing. Dipl.-Gwl. Rocco Eisseler
Group Leader in Machining Technology at the Institute for Maschine Tools (IFW), University of Stuttgart.